Introduction to Attribution – an Overview for Marketing Decision Makers
With endless opportunities to reach potential customers online, brands continue to invest ever larger budgets into digital. But one issue plagues advertisers: how to recognize successful advertising measures? Which campaigns are failing to deliver the desired results and need optimizing? Budgets can only be used efficiently if these questions are answered, and marketing attribution deals with this.
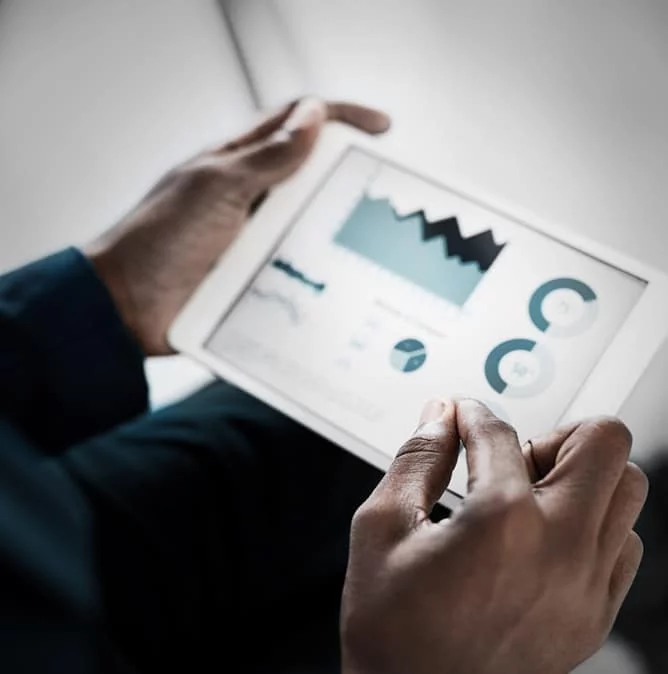
What is marketing attribution?
Marketing attribution is an analysis method that assigns conversions (i.e., the marketer’s goal, such as a product purchase or online registration) to those channels responsible for driving the outcome. Because each purchase results from multiple contact points across different channels, it aims to understand the consumer’s entire purchasing journey. Every touchpoint influences the buying decision, from social media or newsletters to display ads, and marketing attribution’s job is to figure this out.
The different attribution methods
In general, there’s a distinction between rule-based and data-driven attribution.
- Rule-based attribution uses predefined rules and weightings determined by the advertiser. One or more winning touchpoints from the customer journey are selected in advance and assigned a value. This method provides initial insights into a campaign’s effectiveness, but in using fixed rules, it influences the results
- Data-driven attribution is based on objective modeling techniques using algorithms. The algorithm analyses the value of each touch point in the user’s purchase journey and calculates which positively influences the desired conversion. Not only is this more accurate as it’s based on large volumes of data, but it also records the current situation, automatically recognizing any change in the advertising impact. This approach allows advertisers to better plan and optimize their marketing measurement.
Rule-based attribution models
Last touch: In a last touch model, 100% of the credit is given to the last marketing touch point before the conversion. This is still the most widely used attribution model and, as such, is often used as a reference for comparing other attribution models.
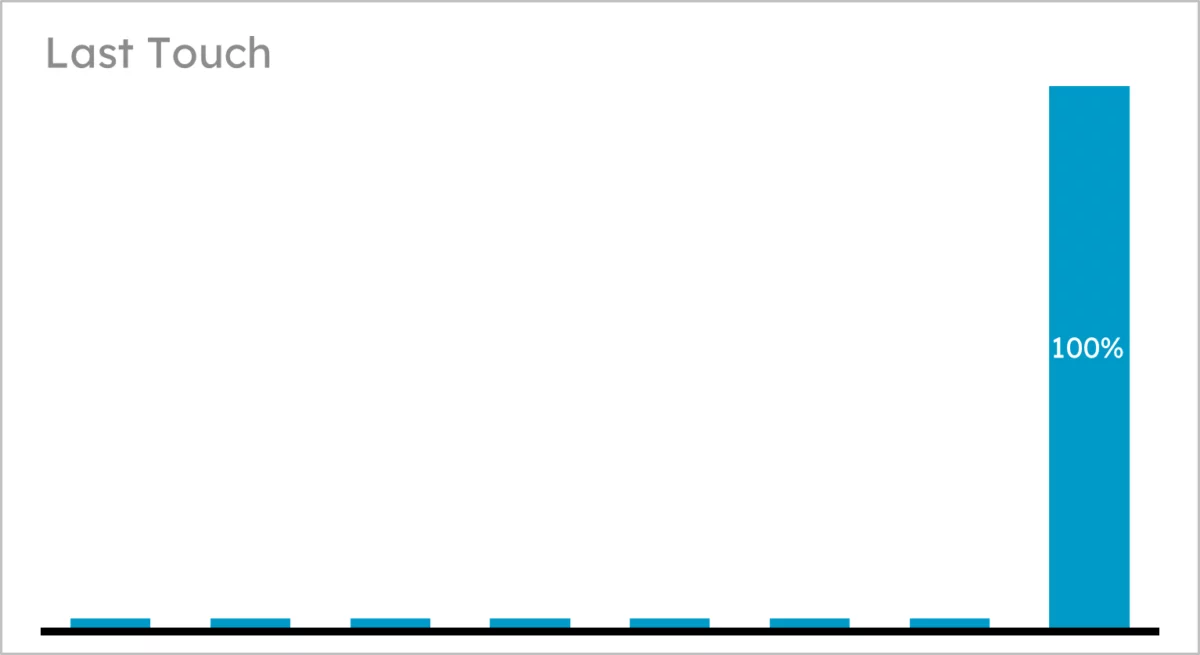
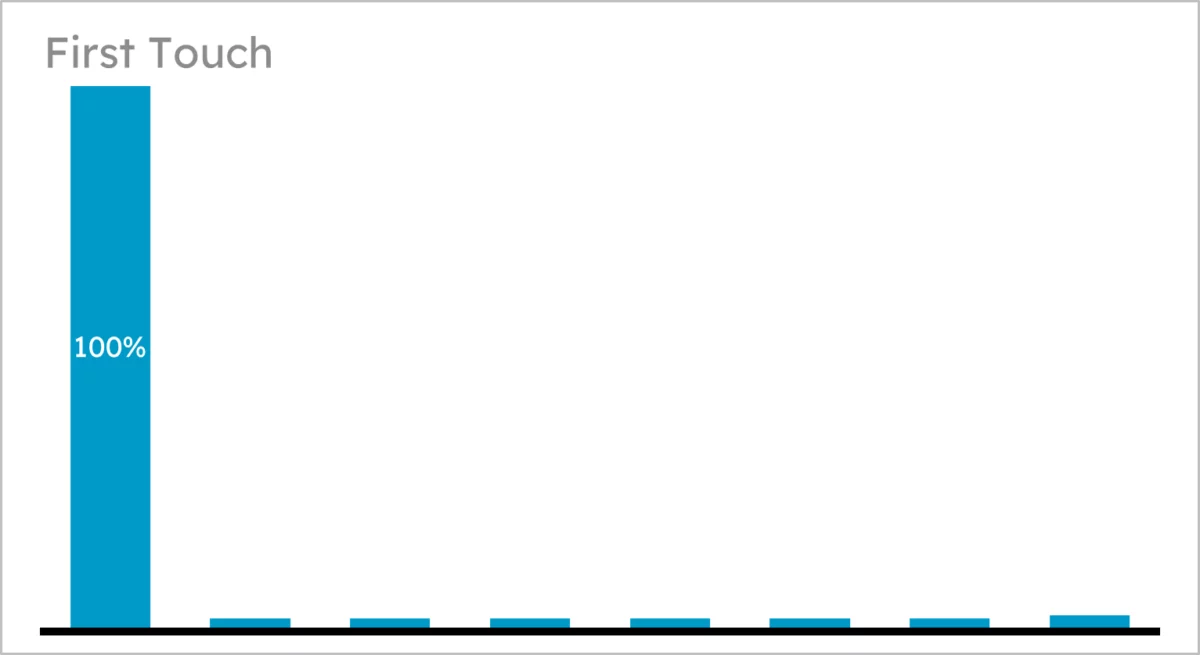
First touch: In a first touch model, 100% of the conversion is credited to the first marketing touchpoint of the customer journey. This is particularly suited for evaluating branding campaigns, as it measures which activity generated the user’s initial awareness.
Linear: In the linear model, each interaction contributing to a conversion is scored equally and assumes every contact point builds on the one before. It’s suitable for awareness campaigns where sales cycles are long as it assumes each customer contact supports brand building.
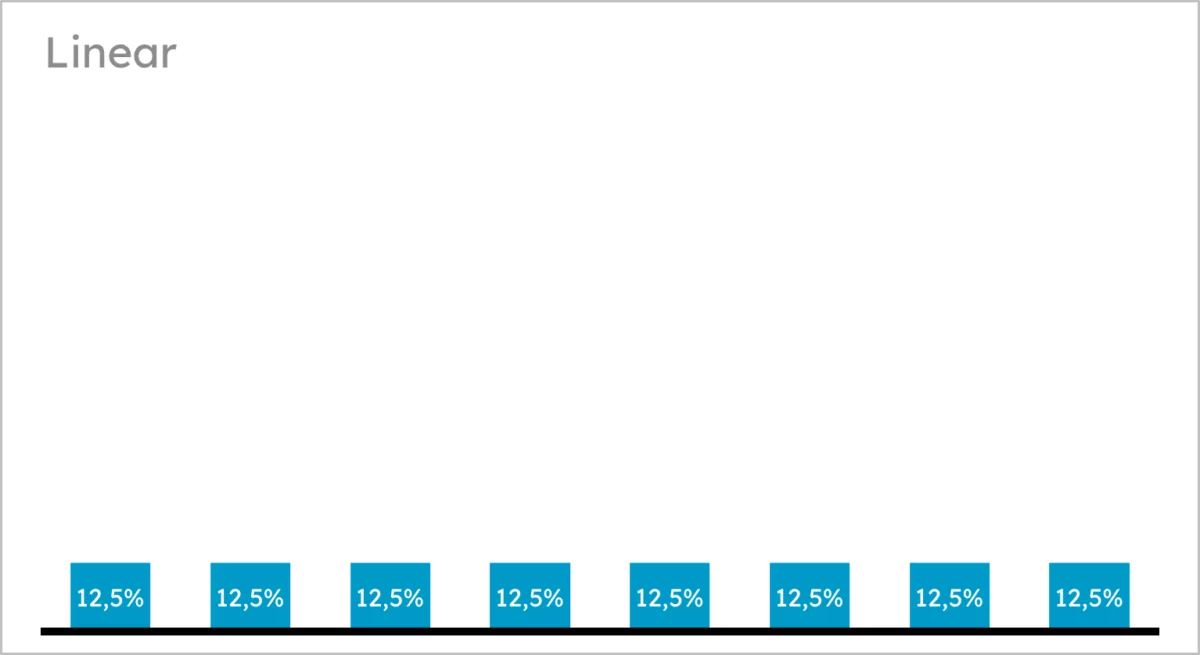
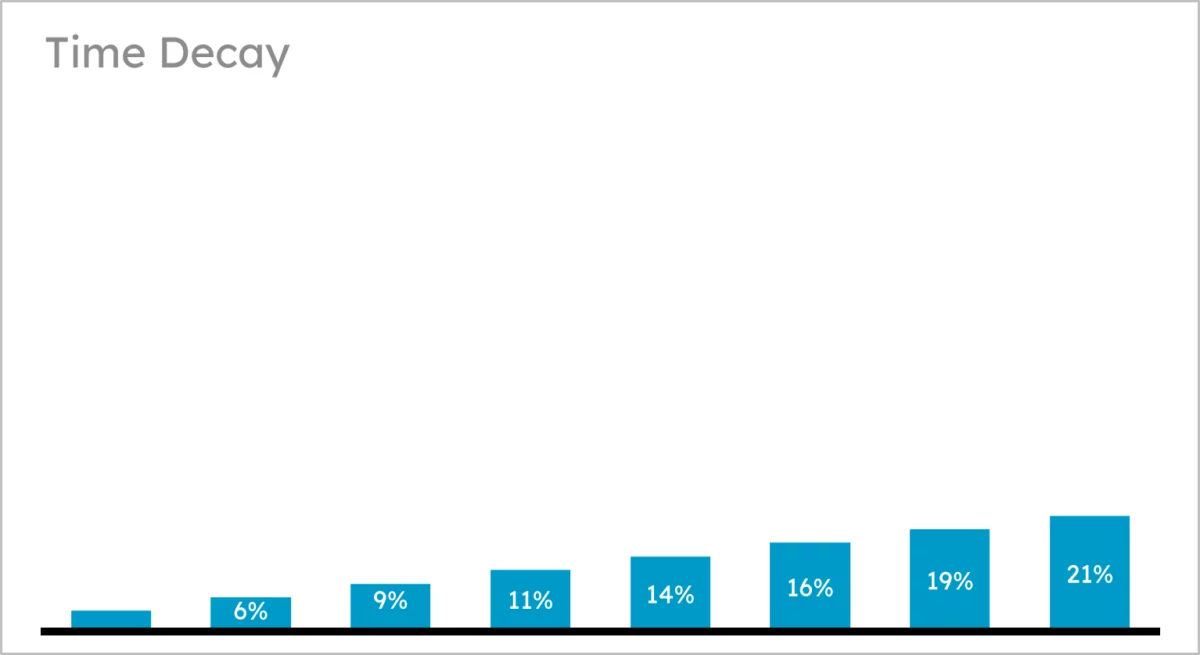
Time decay: With the time decay model, the value of each contact point and the proportion of the conversion assigned to it increases the closer they are to the final conversion. This is relevant where buying decisions are short or for campaigns focused on immediate sales. The model gives weighted credit to those touchpoints closer to the conversion, while “outdated” ones lose value.
Position-based: Also known as a bathtub or U-shaped model, this gives higher importance to the first and last touchpoints in the customer journey, with the others receiving a lower conversion share. A combination of the last touch and first touch models, it’s suitable for advertisers wanting to evaluate their awareness campaigns and the final sale.
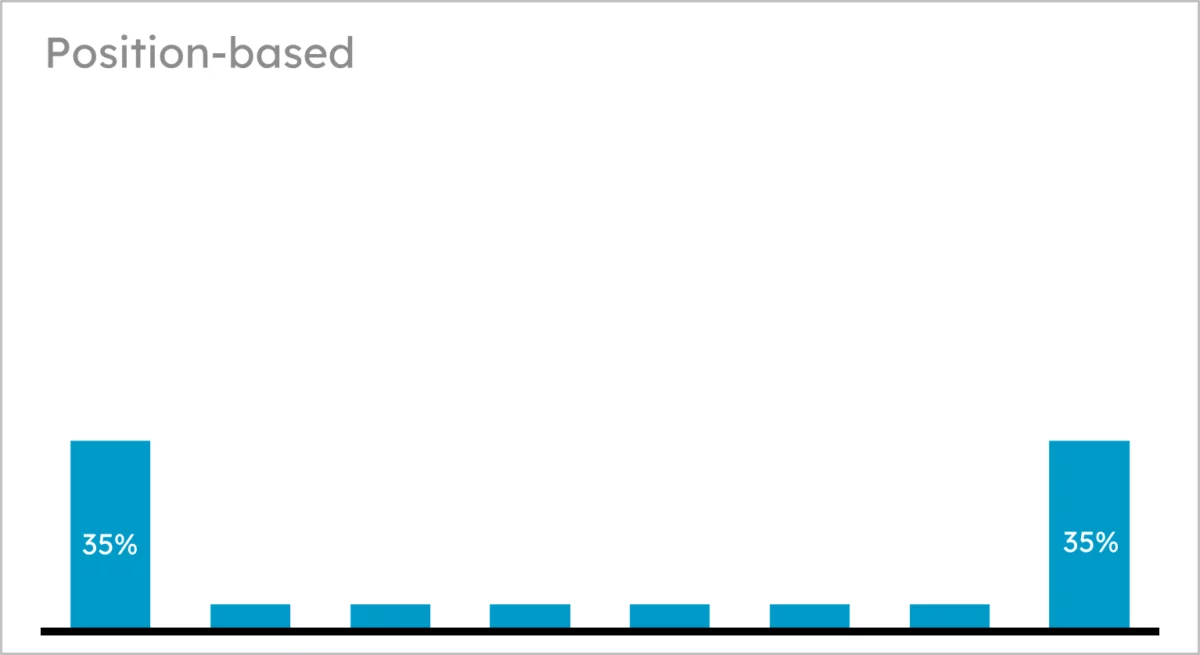
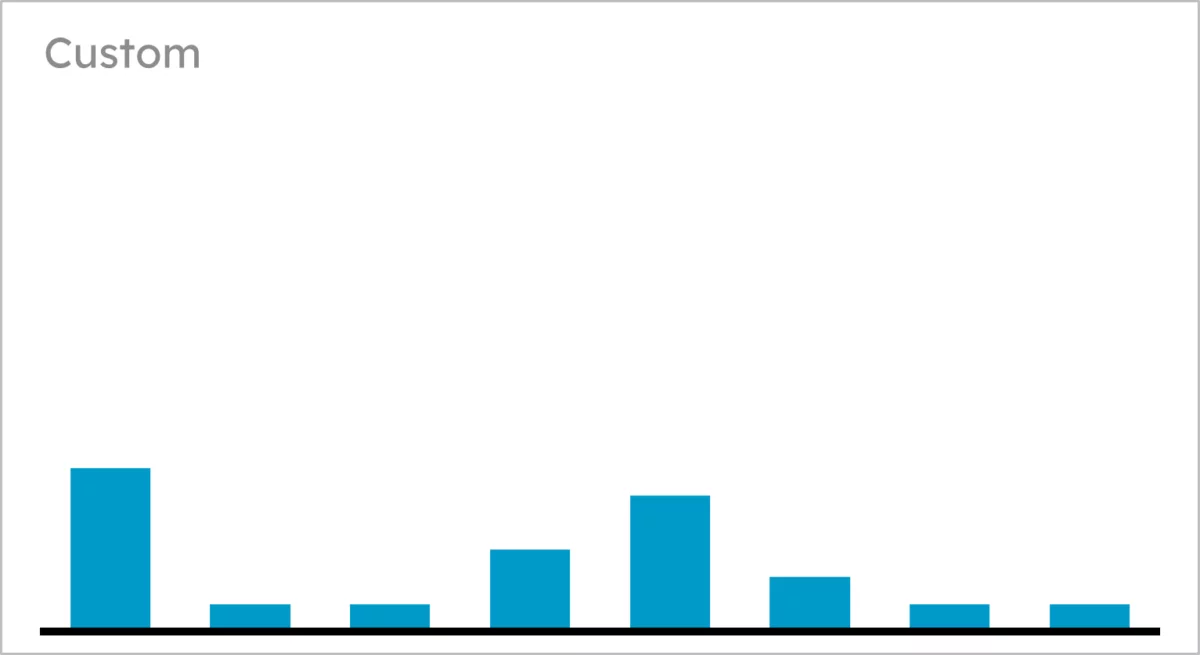
Custom: This is tailored to the needs of the advertiser. Conversion credits can be assigned how the advertiser deems most appropriate for their purchase process.
The different attribution methods
Data-driven attribution determines the importance of each touchpoint based on the accumulated customer journey data. It’s based on algorithms that continuously examine all contact points and calculate which influence the conversion. This approach considers every user interaction, whether successful or not, with those not leading to the desired result being as critical as successful ones. It also considers interactions and synergies between the individual channels and devices, automatically taking into account changes in customer behavior or market conditions.
The key advantage of this model is that each contact point in the customer journey is evaluated objectively. Unlike rule-based approaches, there are no pre-established weightings. Every situation is analyzed to determine each contact point’s contribution to the conversion and is often referred to as a dynamic attribution model.
Another advantage is that sophisticated DDA can cope with non-consented data. Rule-based attribution suffers from data distortion as it relies on user-level, which needs consent. With Exactag’s group-level tracking and DDA advertisers build their attribution on complete data.
Not only can marketers use this to measure their campaign performance objectively, but they can also apply the insights to optimize their future marketing activities and allocate marketing budgets more efficiently across all campaigns.
Which model is the right one?
With a data-driven attribution model, the contribution and influence on a conversion is determined for each touchpoint. This delivers more valid results around the campaign’s impact, so it’s a better approach.
But as compelling as the benefits of dynamic attribution are, it's not for every business. Specific requirements are necessary for the model to deliver to its full potential. For example, data must be available in the volume and quality required for analysis.
If this is not possible, advertisers should continue to adopt a rule-based approach, as any attribution is preferable to taking a siloed approach and evaluating each channel independently. Each rule-based model provides some insight into the customer journey, and the ultimate decision comes down to the business needs the advertiser is pursuing.